Table of Contents
We recently sat down with Sandeep Uttamchandani, Chief Product Officer at Unravel, to discuss the top cloud data migration challenges in 2022. No question, the pace of data pipelines moving to the cloud is accelerating. But as we see more enterprises moving to the cloud, we also hear more stories about how migrations went off the rails. One report says that 90% of CIOs experience failure or disruption of data migration projects due to the complexity of moving from on-prem to the cloud. Here are Dr. Uttamchandani’s observations on the top obstacles to ensuring that data pipeline migrations are successful.
1. Knowing what should migrate to the cloud
The #1 challenge is getting an accurate inventory of all the different workloads that you’re currently running on-prem. The first question to be answered when you want to migrate data applications or pipelines is, Migrate what?
In most organizations, this is a highly manual, time-consuming exercise that depends on tribal knowledge and crowd-sourcing information. It’s basically going to every team running data jobs and asking what they’ve got going. Ideally, there would be a well-structured, single centralized repository where you could automatically discover everything that’s running. But in reality, we’ve never seen this. Virtually every enterprise has thousands of people come and go over the years—all building different dashboards for different reasons, using a wide range of technologies and applications—so things wind up being all over the place. The more brownfield the deployment, the more probable that workloads are siloed and opaque.
It’s important to distinguish between what you have and what you’re actually running. Sandeep recalls one large enterprise migration where they cataloged 1200+ dashboards but discovered that some 40% of them weren’t being used! They were just sitting around—it would be a waste of time to migrate them over. The obvious analogy is moving from one house to another: you accumulate a lot of junk over the years, and there’s no point in bringing it with you to the new place.
How Unravel helps identify what to migrate
Unravel’s always-on full-stack observability capabilities automatically discover and monitor everything that’s running in your environment, in one single view. You can zero in on what jobs are actually operational, without having to deal with all the clutter of what’s not. If it’s running, Unravel finds it—and learns as it goes. If we apply the 80/20 rule, that means Unravel finds 80% of your workloads immediately because you’re running them now; the other 20% get discovered when they run every month, three months, six months, etc. Keep Unravel on and you’ll automatically always have an up-to-date inventory of what’s running.
How Unravel helps cloud migrations
2. Determining what’s feasible to migrate to the cloud
After you know everything that you’re currently running on-premises, you need to figure out what to migrate. Not everything is appropriate to run in the cloud. But identifying which workloads are feasible to run in the cloud and which are not is no small task. Assessing whether it makes sense to move a workload to the cloud normally requires a series of trial-and-error experimentation. It’s really a “what if” analysis that involves a lot of heavy lifting. You take a chunk of your workload, make a best-guess estimate on the proper configuration, run the workload, and see how it performs (and at what cost). Rinse and repeat, balancing cost and performance.
How Unravel helps determine what to move
With intelligence gathered from its underlying data model and enriched with experience managing data pipelines at large scale, Unravel can perform this what-if analysis for you. It’s more directionally accurate than any other technique (other than moving things to the cloud and seeing what happens). You’ll get a running head start into your feasibility assessment, reducing your configuration tuning efforts significantly.
3. Defining a migration strategy
Once you determine whether moving a particular workload is a go/no-go from a cost and business feasibility/agility perspective, the sequence of moving jobs to the cloud—what to migrate when—must be carefully ordered. Workloads are all intertwined, with highly complex interdependencies.
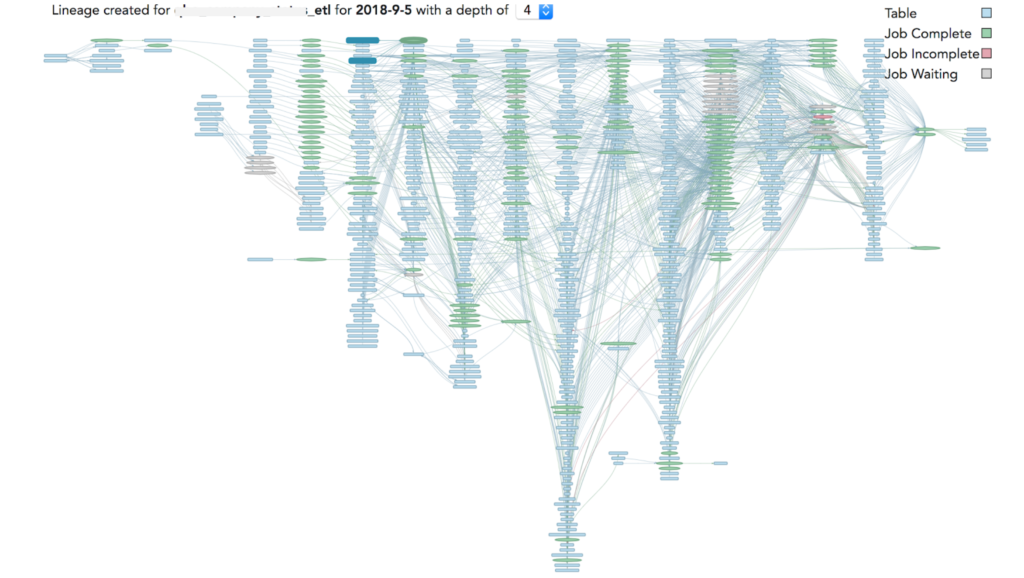
Source: Sandeep Uttamchandani, Medium: Wrong AI, How We Deal with Data Quality Using Circuit Breakers
You can’t simply move any job to the cloud randomly, because it may break things. That job or workload may depend on data tables that are potentially sitting back on-prem. The sequencing exercise is all about how to carve out very contained units of data and processing that you can then move to the cloud, one pipeline at a time. You obviously can’t migrate the whole enchilada at once—moving hundreds of petabytes of data in one go is impossible—but understanding which jobs and which tables must migrate together can take months to figure out.
How Unravel helps you define your migration strategy
Unravel gives you a complete understanding of all dependencies in a particular pipeline. It maps dependencies between apps, datasets, users, and resources so you can easily analyze everything you need to migrate to avoid breaking a pipeline.
4. Tuning data workloads for the cloud
Once you have workloads migrated, you need to optimize them for this new (cloud) environment. Nothing works out of the box. Getting everything to work effectively and efficiently in the cloud is a challenge.The same Spark query, the same big data program, that you have written will need to be tuned differently for the cloud. This is a function of complexity: the more complex your workload, the more likely it will need to be tuned specifically for the cloud. This is because the model is fundamentally different. On-prem is a small number of large clusters; the cloud is a large number of small clusters.
This where two different philosophical approaches to cloud migration come into play: lift & shift vs. lift & modernize. While lift & shift simply takes what you have running on-prem and moves it to the cloud, lift & modernize essentially means first rewriting workloads or tuning them so that they are optimized for the cloud before you move them over. It’s like a dirt road that gets damaged with potholes and gullies after a heavy rain. You can patch it up time after time, or you can pave it to get a “new” road.
But say you have migrated 800 workloads. It would take months to tune all 800 workloads—nobody has the time or people for that. So the key is to prioritize. Tackle the most complex workloads first because that’s where you get “more bang for your buck.” That is, the impact is worth the effort. If you take the least complex query and optimize it, you’ll get only a 5% improvement. But tuning a 60-hour query to run in 6 hours has a huge impact.
How Unravel helps tune data pipelines
Unravel has a complexity report that shows you at a glance which workloads are complex and which are not. Then Unravel helps you tune complex cloud data pipelines in minutes instead of hours (maybe even days or weeks). Because it’s designed specifically for modern data pipelines, you have immediate access to all the observability data about every data job you’re running at your fingertips without having to stitch together logs, metrics, traces, APIs, platform UI information information, etc., manually.
But Unravel goes a step further. Instead of simply presenting all this information about what’s happening and where—and leaving you to figure out what to do next—Unravel’s “workload-aware” AI engine provides actionable recommendations on specific ways to optimize performance and costs. You get pinpoint how-to about code you can rewrite, configurations you can tweak, and more.
Cloud migration made much, much easier
5. Keeping cloud migration costs under control
The moment you move to the cloud, you’ll start burning a hole in your wallet. Given the on-demand nature of the cloud, most organizations lose control of their costs. When first moving to the cloud, almost everyone spends more than budgeted—sometimes as much as 2X. So you need some sort of good cost governance. One approach to managing costs is to simply turn off workloads when you threaten to run over budget, but this doesn’t really help. At some point, those jobs need to run. Most overspending is due to overprovisioned cloud resources, so a better approach is to configure instances based on actual usage requirements rather than on received need.
How Unravel helps keep cost under control
Because Unravel has granular, job-level intelligence about what resources are actually required to run individual workloads, it can identify where you’ve requested more or larger instances than needed. Its AI recommendations automatically let you know what a more appropriately “right-sized” configuration would be. Other so-called cloud cost management tools can tell you how much you’re spending at an aggregated infrastructure level—which is okay—but only Unravel can pinpoint at a workload level exactly where and when you’re spending more than you have to, and what to do about it.
6. Getting data teams to think differently
Not so much a technology issue but more a people and process matter, training data teams to adopt a new mind-set can actually be a big obstacle. Some of what is very important when running data pipelines on-prem—like scheduling—is less crucial in the cloud. That running one instance for 10 hours is the same as running 10 instances for one hour is a big change in the way people think. On the other hand, some aspects assume greater importance in the cloud—like having to think about the number and size of instances to configure.
Some of this is just growing pains, like moving from a typewriter to a word processor. There was a learning curve in figuring out how to navigate through Word. But over time as people got more comfortable with it, productivity soared. Similarly, training teams to ramp up on running data pipelines in the cloud and overcoming their initial resistance is not something that can be accomplished with a quick fix.
How Unravel helps clients think differently
Unravel helps untangle the complexity of running data pipelines in the cloud. Specifically, with just the click of a button, non-expert users get AI-driven recommendations in plain English about what steps to take to optimize the performance of their jobs, what their instance configurations should look like, how to troubleshoot a failed job, and so forth. It doesn’t require specialized under-the-hood expertise to look at charts and graphs and data dumps to figure out what to do next. Delivering actionable insights automatically makes managing data pipelines in the cloud a lot less intimidating.
Next steps
Check out our 10-step strategy to a successful move to the cloud in Ten Steps to Cloud Migration, or create a free account and see for yourself how Unravel helps simplify the complexity of cloud migration.